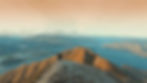
Research Highlights
Let's Build a Better Future.

Adaptive Planning for Urban Stormwater Management
Urban stormwater management is shifting its attention from traditional centralized engineering solutions to a distributed and greener approach, namely Green Infrastructure (GI). However, uncertainties concerning GI's efficacy for reducing runoff and pollutants are a barrier to the adoption of GI. One strategy to deal with the uncertainty is to implement GI adaptively, in which stormwater managers can learn and adjust their plans over time to avoid undesired outcomes. We propose a new class of GI planning methods based on two-stage stochastic programming and Bayesian learning, which accounts for projected information gains and decision makers' objectives and willingness to accept risk. In the hypothetical example, the model identifies four categories of investment strategies and quantifies their benefits and costs: all-in, greedy investment plus deferral, mixed investments plus deferral, and learn-and-adjust. Which strategy is optimal depends on the user's risk attitudes, and the alternatives' costs and risks.

Water Scarcity and Human Adaptation
One major challenge in water resource management is to balance the uncertain and nonstationary water demands and supplies caused by the changing anthropogenic and hydroclimate conditions. To address this issue, we developed a reinforcement learning agent-based modeling (RL-ABM) framework where agents (agriculture water users) are able to learn and adjust water demands based on their interactions with the water systems. The intelligent agents are created by a reinforcement learning algorithm adapted from the Q-learning algorithm. We illustrated this framework in a case study where the RL-ABM is two-way coupled with the Colorado River Simulation System (CRSS), a long-term planning model used for the administration of the Colorado River Basin, for assessing agriculture water use impacts on water scarcity. Seventy-eight intelligent agents are simulated, which can be grouped into three categories based on their parameter values: the “aggressive” (swift actions; low regrets), the “forward-looking conservative” (mild actions; high regrets; fast learning), and the “myopic conservative” (mild actions; median regrets; slow learning). The ABM-CRSS results showed that the major reservoirs in the Upper Colorado Basin might experience more frequent water shortages due to the increasing water uses compared to the original CRSS results. If the drought continues, the case study also demonstrates that agents can learn and adjust their demands.

Green Infrastructure for Combined Sewer Overflows Control
We investigate how the effectiveness of green infrastructure (GI) to mitigate the frequency and magnitude of significant discharge events and combined sewer overflows (CSOs) depend on both climate and sewershed characteristics and propose a theoretical framework for a holistic assessment of GI's efficacy. The framework is based on the comparison of three characteristic timescales that control the production of peak discharge: rainfall duration (tr), travel time in the sewer network (tn), and the duration of rain that would be required to fill the GI's storage (tGI). Storm events can then be characterized by two ratios of these timescales: Tn = tn/tGI and Tr = tr/tGI. A third dimensionless number characterizes critical storms during which adverse events (such as CSOs) occur and allows us to identify the combinations of Tn and Tr for which GI may substantially mitigate those events. The results of numerical experiments with the model demonstrate that the storms for which GI can substantially reduce peak discharge and CSO volume typically occur in a narrow band of Tn and Tr. Within that band, the efficacy of GI may depend on the location of GI within the sewershed if network routing substantially affects the timing and magnitude of flood peaks. The proposed framework is applied to examine the efficacy of GI using historical precipitation data from two major U.S. cities: Philadelphia, PA, and Seattle, WA, and the results of this comparative analysis suggest that GI location is an important control on catchment-scale GI efficacy in Philadelphia, but less so in Seattle.